Big Data in Catastrophe and Supply Chain Risk Modeling
As “big data” quickly becomes the new standard for “all data” in technology-driven enterprises, advanced data analytics has become the key to competitive advantage in a number of fields, including supply chain risk modeling and mitigation. However, deriving actionable insights from these multi-gigabyte or petabyte-sized datasets with mixed multi-dimensional variables isn’t easy. For those organizations still early in their journey to become data-driven, it can be helpful to look at how professions that have long relied on immense databases to make informed decisions, such as climate scientists, seismologists, meteorologists and risk modelers, are utilizing these datasets to manage and mitigate risk.
Government agencies, including the United States Geological Survey (USGS) and the National Oceanic and Atmospheric Administration (NOAA), for example, have been collecting and analyzing climatological and geological data for over a century. They have amassed an incredible cache of historical data on a range of catastrophic events that pose a significant threat to human lives and property, including hurricanes, earthquakes, coastal storm surges, tsunamis and riverine flooding. With recent advances in computing power, these agencies can now assimilate this complex data more rapidly and accurately, providing consumers and businesses the insights they need to make more informed decisions to improve safety and reduce financial losses. For example, the USGS PAGER1 system analyzes large earthquakes in near real-time to generate damage and casualty estimates that government agencies, aid organizations, and corporations can use to rapidly assess the impact of an event.
For those still early in their journey to become data driven, it can be helpful to look to professions, like seismology and meteorology, that have long relied on extensive databases to make informed decisions.
Risk modelers can leverage the same data and extend its value through the use of catastrophe risk models, which output detailed financial loss and supply chain interruption estimates. For example, following the April 2016 M7.0 earthquake that struck the Kumamoto and Oita prefectures in Japan, AIR Worldwide issued an assessment of the global impacts to the automotive supply chain, which identified impacted corporations and provided stakeholders in the automotive and insurance industries with direct and contingent business interruption loss estimates2. More generally, catastrophe risk modeling companies use these high-resolution data products to build detailed risk models that enable corporations and re/insurers to gain a more accurate view of their risk and devise better business continuity strategies.
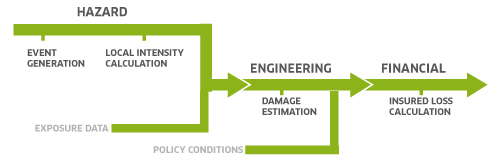
Figure 1
At AIR Worldwide3, we build catastrophe risk models by combining historical data on catastrophic events with state-of-the-art simulation methods to generate a probabilistic perspective of current and future risks. These models, which leverage vast quantities of hazard and asset data, play a critical role for corporate and re/insurance companies in terms of quantifying and mitigating their property and supply chain risk due to natural and manmade catastrophic events. Unlike the deterministic or “what-if” methods employed in traditional supply chain risk assessments, the catastrophe risk models use an expansive catalog of possible supply chain disruption scenarios to provide supply chain managers a probabilistic view of their supply chain risk. Figure 1 illustrates the general framework of a catastrophe risk model. A brief overview of different components of the model, along with the associated data, is provided in the following paragraphs.
Exposure: The exposure data is a collection of physical and engineering attributes of an insured asset. For example, the supply chain exposure dataset would include the physical and geospatial details for both suppliers and a company’s production facilities. In addition, the exposure could contain historical supply chain disruption information at each of the supplier locations, with the associated insurance premium and claims data. Exposure datasets additionally can include product codes supplier tier information, and mitigation measures (e.g. Reserves, Redundancy, and Resilience) adopted by each supplier.
Hazard & Damage Estimation: For each supplier location in the exposure dataset, the hazard module calculates the hazard intensity (e.g. earthquake shaking, hurricane wind speed, flood depth, etc.) for both deterministic (i.e. historical) and stochastic (i.e. possible future) events. Damage functions, which use claims data, post-event surveys, and engineering analysis to relate hazard intensity to physical damage, are evaluated at each supplier location to assess the likelihood of a supply chain disruption. Using supply chain product flow network information, the disruption at one supplier is propagated to the downstream supplier(s). As a measure to avert risk, a corporation may use the hazard data during its supplier selection process to eliminate suppliers in high-risk regions or to formulate mitigation measures to protect against potential supply chain disruptions.
Catastrophe risk models combine historical data on catastrophic events with state-of-the-art simulation methods to generate a probabilistic perspective of current and future risks.
Financial: In addition to physical damage and direct business interruption losses for individual suppliers, the financial module calculates contingent business interruption losses due to historical and stochastic supply chain disruption events. The supply chain loss estimates may be outputted as either Business Interruption (BI) in days or as an equivalent Product Value at Risk (PVaR), which represents lost revenue from production shortfalls. Using the loss estimates, critical suppliers and products can be identified, which in turn can aid corporations in planning for contingencies by either buying appropriate insurance cover or developing mitigation measures with their suppliers.
Catastrophe risk models offer critical risk metrics and insights that can be integrated into a corporate supply chain risk management strategy. To fully harness the value of risk models, organizations must close the gaps that exist in their collection and management of supply chain data. To this end, developing and implementing a supplier “data standard” that houses information about each supplier’s facility, products, and historical disruptions, is an effective first step towards capturing the inherent vulnerabilities in a supply chain network. Additionally, data that is already available in an organization’s ERP systems can be analyzed to detect correlations between past events and supply disruptions, providing a retrospective method for identifying critical suppliers and network deficiencies. Organizations that are capable of combining their existing data with advanced risk modeling tools will achieve best-in-class risk mitigation and move towards identifying their prospective drivers of supply chain failure before a disruption ever occurs.
1 http://earthquake.usgs.gov/data/pager/
2 http://w3.air-worldwide.com/SupplyChainIssueBriefRegistration
3 www.air-worldwide.com